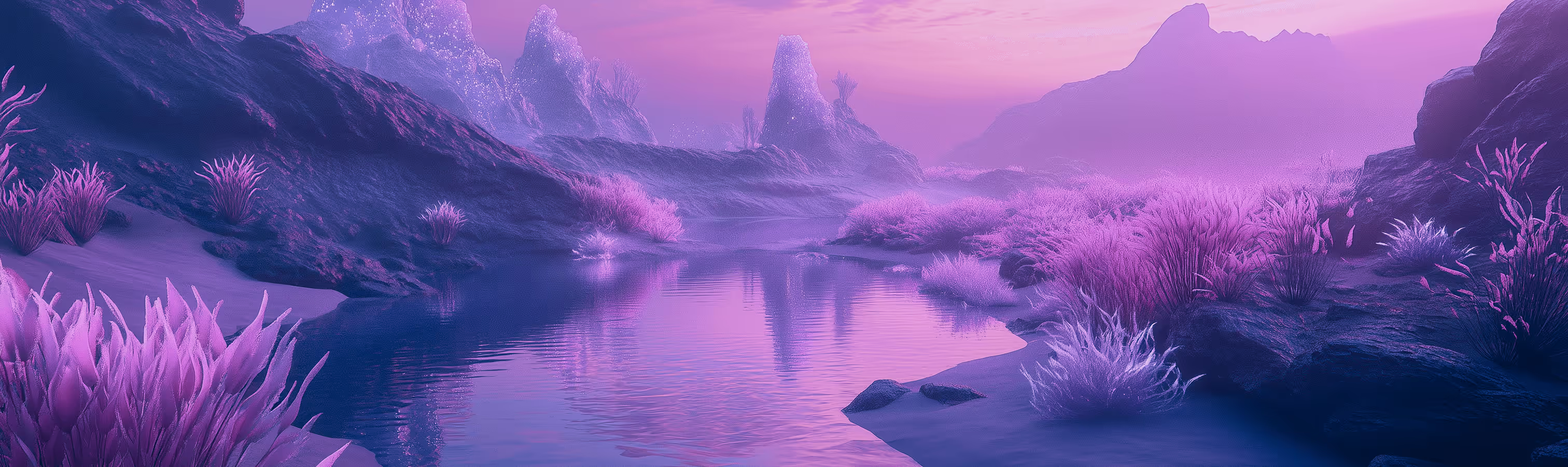
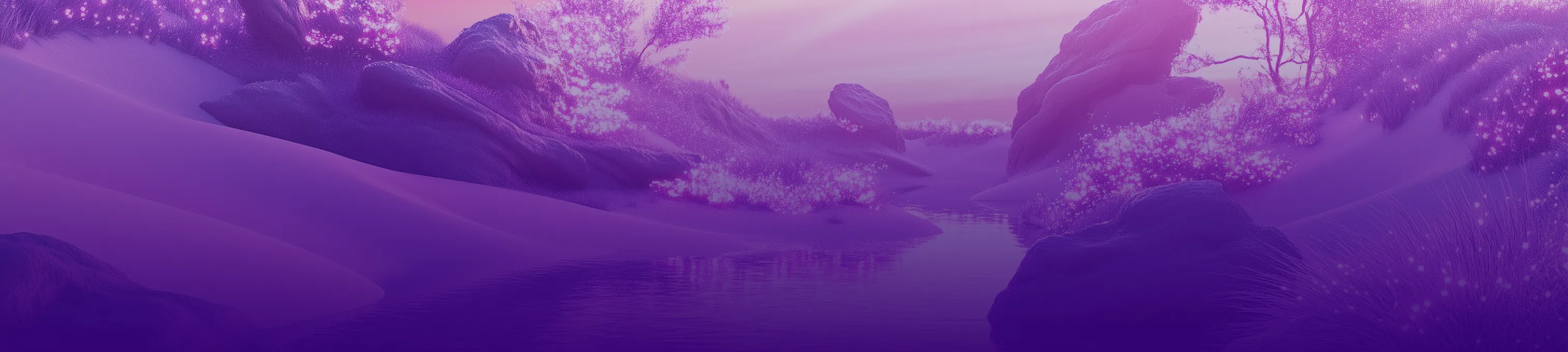
Revolutionizing AI Fine-Tuning with the 0G Compute Network
AI models are pretrained on vast, generalized datasets: books, websites, code repositories, and more. That’s how they learn grammar, syntax, and reasoning. But pretraining only gives you general intelligence, not a specialist. If you want a model that can conduct biotech research or write in your brand’s voice, this will require fine-tuning: the process of retraining a base model on a smaller, domain-specific dataset to adapt it to a new task.
As AI models become more ubiquitous and sophisticated, the demand for secure, cost-effective, and high-performance fine-tuning solutions has skyrocketed. Traditional approaches typically rely on centralized providers or fragmented infrastructures. However, these methods often lack transparency, can limit flexibility, and may lead to uncertainty around costs and data privacy.
Enter the 0G Compute Network—an evolving ecosystem designed to bring customers and providers together under a single, trustless, and verifiable framework for fine-tuning (and in the future, additional AI services).
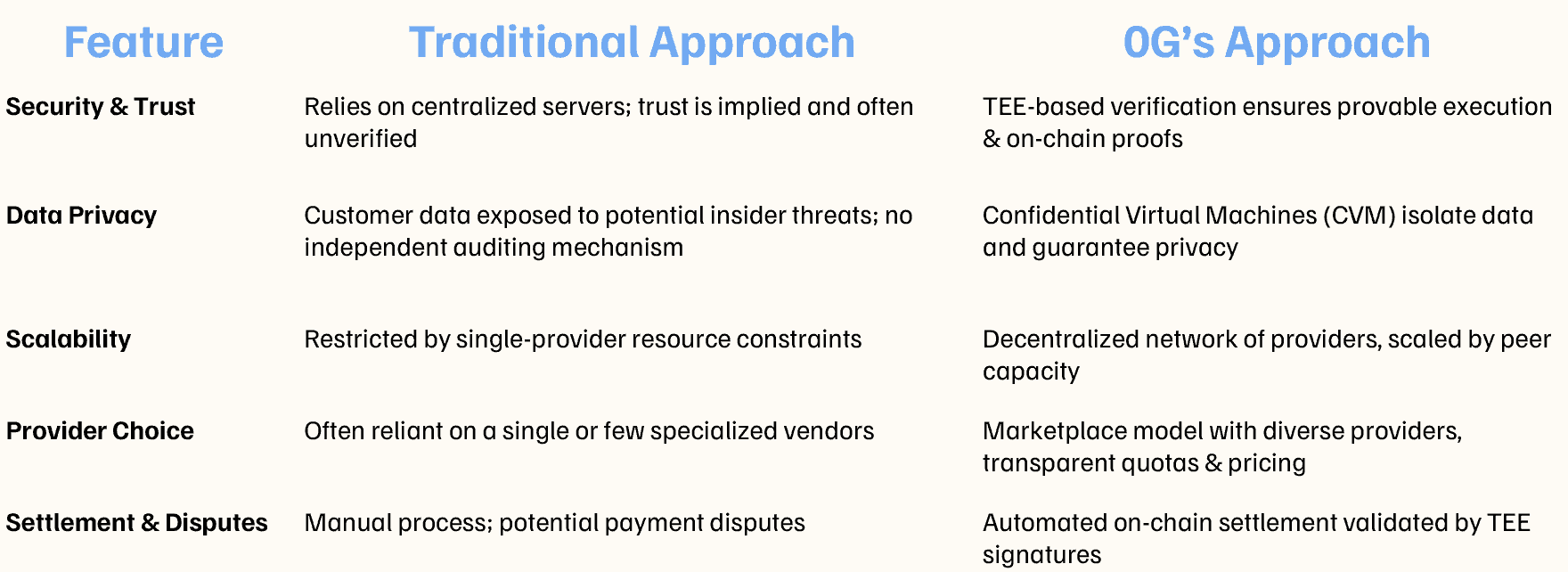
The result is a programmable, open system that empowers secure, efficient, and profitable model training at scale. Let’s unpack how these features converge to create a cutting-edge network for fine-tuning AI models.
Architectural Overview
The 0G Compute Network’s integrated fine-tuning framework redefines how customers and providers collaborate in AI model training. By blending trusted execution environments, robust on-chain settlement, and flexible orchestration, we are creating a platform where:
- Customers can confidently upload data and scripts, pay only for the services used, and retrieve models securely.
- Providers can offer transparent, profitable, and scalable services without losing time chasing down payments.
Below is a high-level representation of how fine-tuning is orchestrated within the 0G Compute Network:
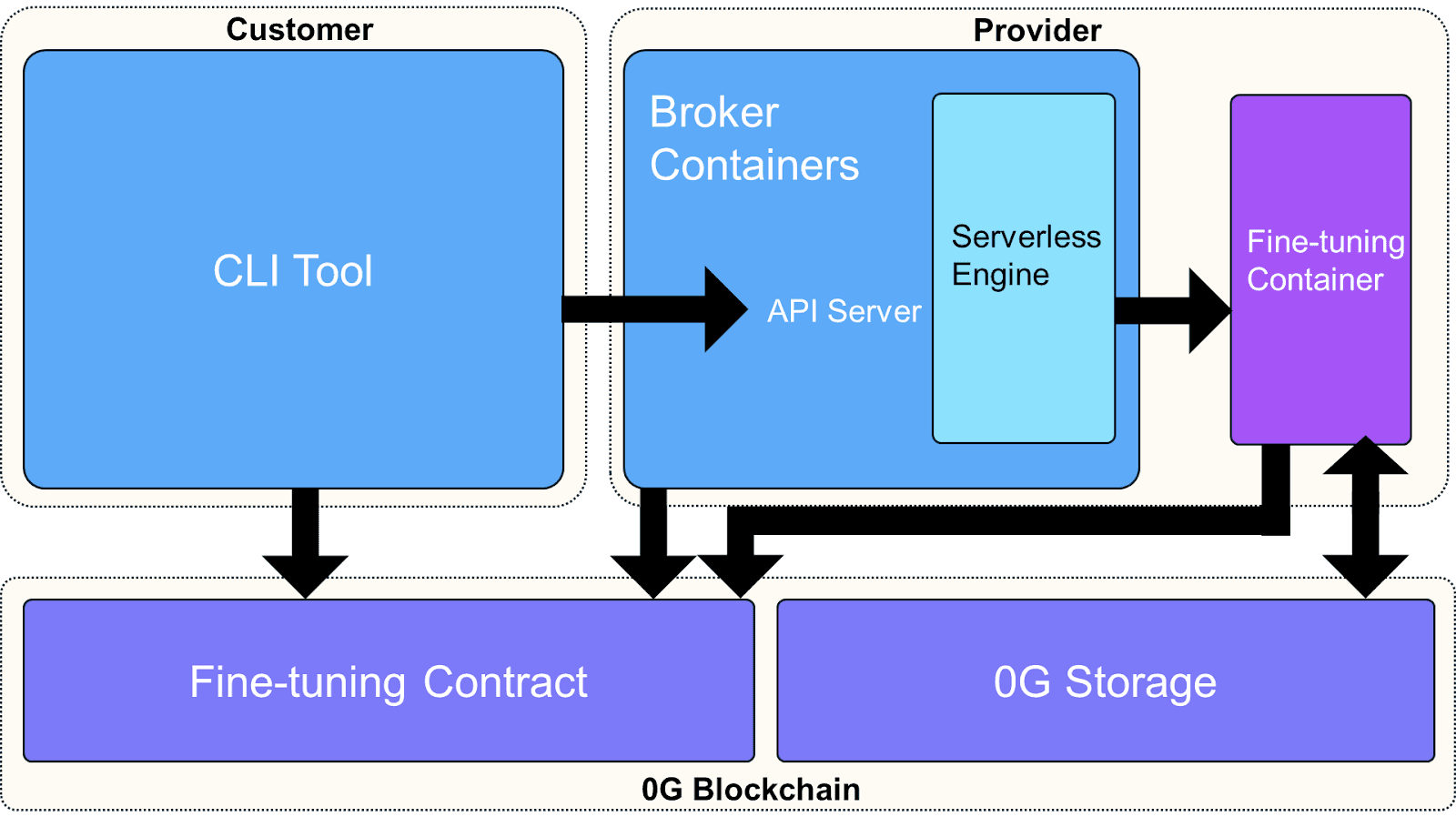
How It Works
1. Providers Offer Secure Fine-Tuning Services
Providers launch their servers in a Confidential Virtual Machine (CVM) and publish their fine-tuning offerings on the 0G Compute Network. Each server generates a signing key pair at startup, which becomes integral for verifiable, on-chain proof of execution. Customers can download a Remote Attestation (RA) report to verify that the provider is running securely within a TEE. This ensures both parties have complete confidence in the authenticity of any subsequent computations.
Key Takeaways for Providers
- Providers can customize quotas (CPU, memory, GPU) and price structures.
- The TEE-based system and on-chain contracts protect provider fees and profitability.
- Only one fine-tuning task can run at a time, preventing resource over-allocation and performance drops.
2. Customers Verify and Fund Tasks
Customers verify a provider’s identity using the RA report and signing public key. They create an account in the 0G contract, depositing initial funds. This ensures that the cost of any subsequent task is covered, establishing a straightforward pay-as-you-go model.
Key Steps for Customers
- Create an account on the contract with the customer’s wallet key
- Pre-deposit funds for future tasks.
- Download & verify the RA report from the desired provider.
3. Task Creation & Execution Occurs Through 0G
When customers are ready to fine-tune a model, they craft a signed task request specifying parameters such as the dataset hash, pre-trained model hash, fine-tuning script hash, and agreed-upon fee. The provider then executes the fine-tuning job inside a secure environment and signs the results with its TEE keys.
Execution Process Highlights
- Signature Verification: The provider validates the task request, ensuring correctness and sufficient customer balance.
- Dataset & Model Check: The provider downloads and verifies the model, dataset, and script from 0G Storage.
- Fine-Tuning: A specialized script (provided by the 0G team or preset by the provider) runs the training process.
- Encryption & Upload: Results are encrypted, securely stored on 0G Storage, and hashed for on-chain references.
- TEE-Signed Settlement: The provider generates a TEE signature with final metadata (model root hash, encrypted secret, fees, etc.).
4. Secure Retrieval & Settlement
Once the job is completed, the encrypted model is made available on 0G Storage. The customer downloads it, confirms the integrity of the file using its root hash, and calls the contract’s /acknowledge function. At this point, settlement triggers, transferring the previously agreed-upon fee to the provider. The customer then decrypts the model using a key that the provider stored on the contract during the settlement process. This key is encrypted with the customer's public key, ensuring that only the customer can decrypt the model.
Why It Matters
- No Payment Surprises: Fees are locked upfront and released only upon successful completion and verification.
- Customer Control: Customers confirm they’ve received the expected model before payment is finalized.
- Provider Protection: On-chain settlement with TEE-proven authenticity eliminates disputes and ensures fair compensation.
Contracts: Enabling Trust & Transparency
At the heart of the 0G Compute Network is a modular trio of smart contracts, purpose-built to encode financial, operational, and reputational logic around fine-tuning tasks:
- serving: serves as the main entry point and execution hub for creating tasks and settling payments.
- account: manages user balances, nonces, and public keys. Tracks deliverables so customers can easily retrieve their models.
- service: stores provider details like quotas, pricing, and general service operations.
Together, these contracts offer a programmable substrate for trustless collaboration. Customer-provider trust is encoded as logic and enforced at the protocol level, meaning all involved parties have granular control over financial transactions and can audit each step of the fine-tuning lifecycle.
Fine-Tuning Fee Calculation
The fee model for fine-tuning is simple but principled: you pay for what you use. That includes:
- Dataset size
- Number of epochs
- GPU price (set by providers)
- Storage costs for 0G Storage
This model aligns incentives clearly and fairly. Customers can optimize their costs by tuning only what’s necessary, while providers can differentiate based on compute throughput, efficiency, and availability. And since all fees are held in escrow and only released upon verifiable delivery, both sides avoid ambiguity or risk.
Customer Experience: Command-Line Interface (CLI)
To make this infrastructure accessible, the 0G has built a purpose-built command-line interface (CLI) that serves as a frontend to the protocol’s logic layer. CLI commands include:
- add-account
- deposit-fund
- create-task
- get-log
- download-model
The CLI also automatically handles file uploads to 0G Storage, root hash retrieval, and secure downloads. This one-stop tool enables customers to manage the entire lifecycle of their fine-tuning tasks, from account creation to final model decryption.
Provider Experience: Execution & Broker Images
On the provider side, we offer a Provider Execution Image for secure script execution and a Broker Image for receiving, scheduling, and managing tasks. The broker:
- Validates incoming requests against the provider’s resource limits.
- Forwards tasks to a Serverless engine for containerized execution.
- Tracks task states and ensures only one fine-tuning job runs at a time on each provider.
- Integrates with the CVM interface so the provider can offer verifiable services without managing implementation details.
A Unified Network: Fine-Tuning & Chatbot Services
The 0G Compute Network doesn’t stop at fine-tuning. It’s designed to support an entire ecosystem of AI services within one unified protocol. In other words, while fine-tuning and inference involve distinct internal processes, both will be unified under the 0G Compute Network, enabling:
- Shared Account System: A higher-level parent contract can manage user balances across multiple child accounts, ensuring each provider is compensated independently without the user juggling multiple balances.
- Single Point of Interaction: Customers can tap into a consolidated interface—CLI or SDK—to seamlessly switch between different services (fine-tuning, chatbot inference, etc.).
By consolidating these services, users benefit from a cohesive experience and providers gain exposure to a broader client base while retaining autonomous control over pricing and configurations. This reduces onboarding time for new services, and creates a seamless experience for developers looking to build, deploy and scale powerful AI applications within one unified environment.
Beyond Fine-Tuning
Fine-tuning is just the beginning. 0G is evolving into a full-stack operating system for decentralized AI, and we will continue to refine our infrastructure and user interfaces. The team is actively working to expand the ecosystem with more robust features, including:
- Enhanced Preset Models: Providers can publish standard or custom pre-trained models for quick integration.
- Extended Contract Functionality: Additional settlement methods, partial refunds, and more advanced billing cycles.
- Developer SDKs: Beyond CLI, an SDK is in progress to give developers programmatic access for building integrated AI workflows.
These developments will further empower everyone from research labs to enterprise-grade ML teams, as they unleash the next wave of fine-tuned intelligence on 0G.
A special thanks to the Phala team, whose work in TEE, Confidential Virtual Machines (CVM), and verifiability techniques has greatly supported the 0G Compute Network. Their contributions enable high-level security guarantees and transparent execution, ensuring that all parties can trust 0G’s computational integrity and authenticity.